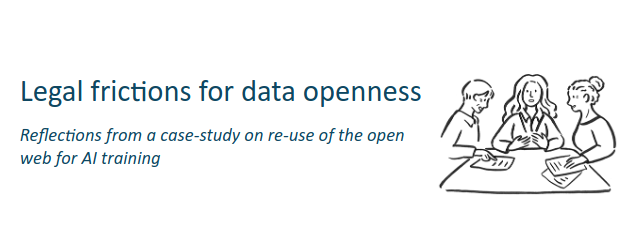
Legal frictions for data openness: Reflections from a case-study on re-use of the open web for AI training
In 2024, inno³ and Open Knowledge Foundation provided support to legal researcher Ramya Chandrasekhar (CIS-CNRS) for her research project on the use and reuse of data from the open web to build foundation AI models.
In 2024, inno³ and Open Knowledge Foundation provided support to legal researcher Ramya Chandrasekhar (CIS-CNRS) for her research project on the use and reuse of data from the open web to build foundation AI models. A report published at the beginning of 2025 draws some conclusions about the current controversies surrounding the use of publicly accessible data to develop AI models, and the role of openness in these debates.
The research project ODECO, which began in October 2021 and ran for 4 years, was designed to examine the open data environment on a European scale. Within this framework, Ramya Chandrasekhar was involved in ‘ESR3 – Value assessment and (re-)distribution in sustainable ODECO’, which focuses on the valorisation and re-use of open data to create new models. As part of a case study on training data for Artificial Intelligence (AI) models, she worked jointly with inno³ to better understand the use of publicly accessible data in these foundation models.
She analysed various issues with the use of this data within these foundation models and studied current solutions/initiatives to tackle data extractivism that is not respectful of people and cultures.
Project Presentation
The functioning of AI, in particular generative AI models and LLM (Large Language Model) models, relies above all on training data from the open web. This data can be of various types: open data accessible under an open licence or in the public domain, public data and data available under certain conditions.
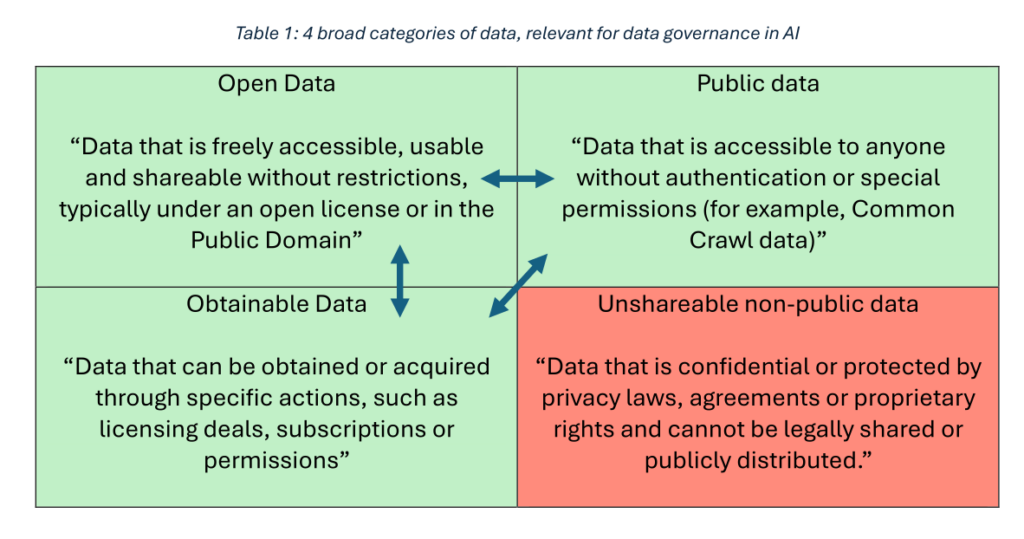
This report focuses on “foundation models” – i.e. AI models that rely on large amounts of training data, as well as large quantities of computational resources for processing this training data.
Definition from the report
Using this data to train AI models is challenging. Firstly, it is difficult to keep track of the data flow. How is it reused, where and why? It is complex to identify the origin of the data once it has been assembled, for example to develop an AI model.
Secondly, data flows are considered to be ‘traceable, stable and contained’ from legal and political points of view in the context of AI, but in reality, the reuse of data is an ‘inherently entangled phenomenon’.
Methodology and research phases
To study the above issues, the first phase of the research was to analyse a series of legal controversies relating to copyright and the protection of training data.
At the same time, Ramya conducted a series of semi-structured interviews with AI researchers and practitioners in Europe and North America. A workshop was then held with users of AI models, researchers, and policymakers from different regions (Europe, USA, UK, Sub-Saharan Africa) to complement these interviews.
At the same time, a set of open and permissive licences was analysed, as well as alternative licences addressing AI-related issues, in order to understand their relationship to open data. This research yielded a comprehensive list of controversies, focusing on international cases like the United States, Europe, the United Kingdom, India, Brazil, Canada and China.
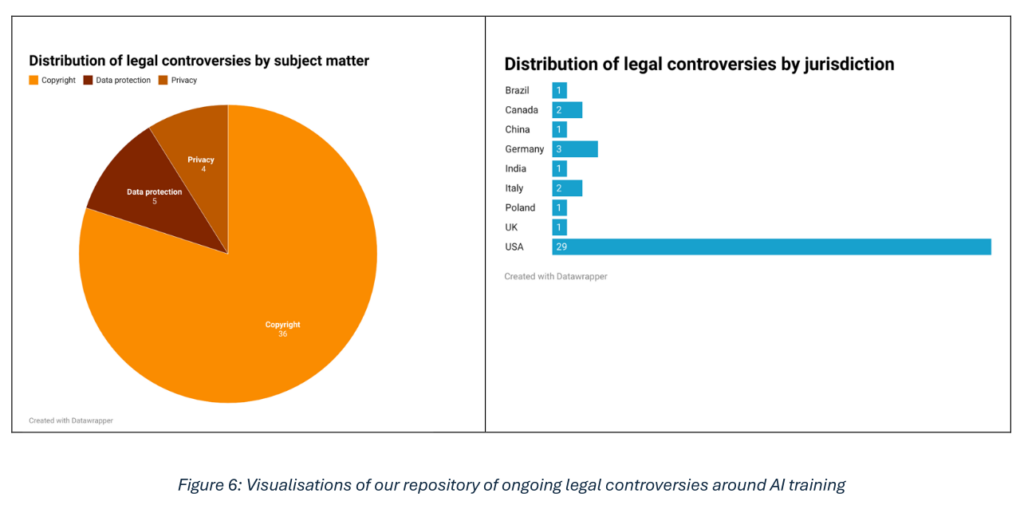
Main results and content produced
The report clearly highlights a number of tensions between data flow modelling and the development of AI models. Here are the key points:
- The construction of a three-dimensional framework for understanding the challenges of openness in the context of training data for AI models,
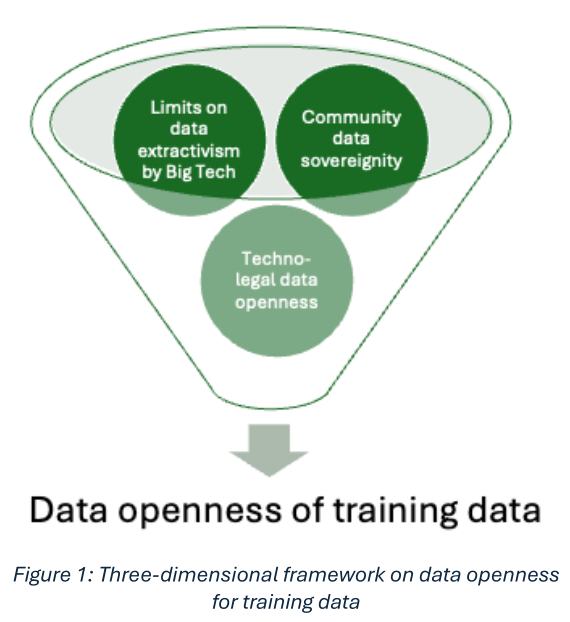
- A list of 41 ongoing legal controversies concerning copyright and the protection of training data for AI models,
- A critical analysis of existing open source licences, permissive licences and alternative licensing frameworks for AI model training data.
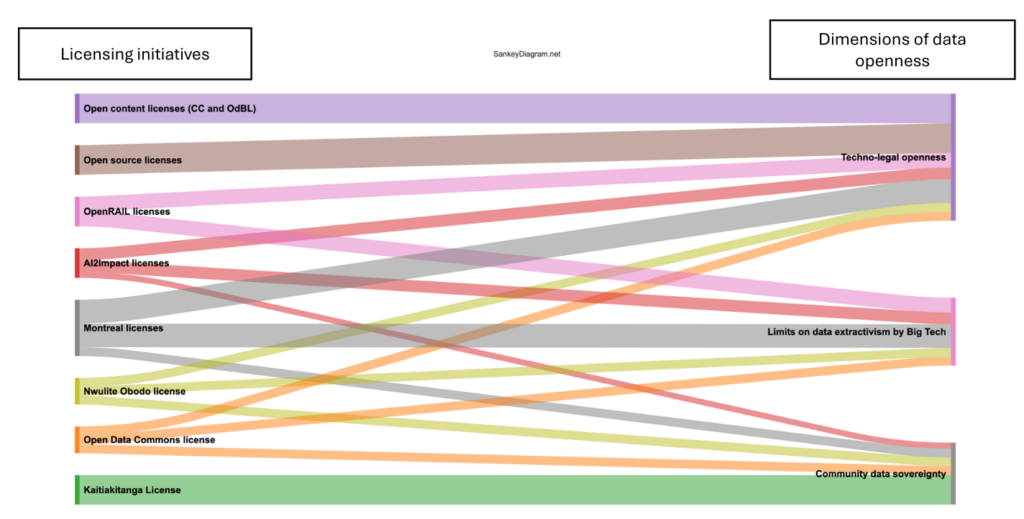
The report is available on the French national archive HAL under a CC-by-SA 4.0 licence: https://hal.science/hal-05009616
To cite the report : Ramya Chandrasekhar. Legal frictions for data openness: Reflections from a case-study on re-use of the open web for AI training. Centre Internet et Société – CNRS; Inno3; Open Knowledge Foundation. 2025, pp.75. ⟨hal-05009616⟩